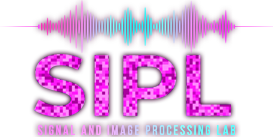
Today, the challenges of security and information safety are substantial, necessitating the development of high-quality and reliable verification methods. The use of biometric authentication methods is expanding as they provide secure and convenient means of verification. In this project, we explore the potential of using the PPG signal as a unique biometric authentication method. This signal represents changes in blood volume during cardiac cycles. Each individual’s PPG signal is influenced by a unique combination of physiological characteristics. This uniqueness allows us to use the PPG signal as a sort of "fingerprint" to identify the person. One significant advantage of PPG-based authentication is that most modern smartwatches and wearable devices incorporate PPG sensors on the wrist. These devices use PPG measurements for heart rate monitoring, but this data can also be used for authentication purposes. This approach enables quick, convenient, and cost-effective authentication without restricting user mobility or requiring active user involvement. Additionally, continuous authentication can be performed over extended periods. In this project, we have developed a deep learning-based model for PPG signal-based authentication. The model demonstrates successful authentication across a large dataset of over 4,400 subjects. Moreover, our model does not require retraining when new subjects are added to the database, which is a significant advantage for practical applications.