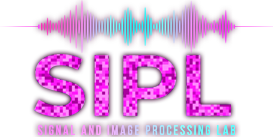
In 2020, the COVID-19 pandemic, a highly contagious respiratory disease, began spreading globally. Before effective vaccines were developed, the primary strategy to control the outbreak was to quickly identify and isolate infected individuals to prevent them from transmitting the virus to others. To facilitate this, we sought in this project to develop a tool that could efficiently detect the presence of the coronavirus through analysis of patient cough sounds recorded on smartphones. A major challenge was obtaining relevant training data, so we used data from similar projects conducted at other universities. We trained several neural network models to perform binary classification of the cough sounds as either positive or negative for COVID-19. However, the results were ultimately unsatisfactory. The likely reason for this is that cough sounds alone do not contain enough differentiating information to reliably distinguish COVID-19 from other respiratory illnesses.