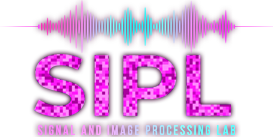
Cancer is a leading cause of death worldwide, responsible for around ten million deaths in 2020. Cancer cells exhibit invasive behavior, which significantly contributes to tumor growth and metastasis. The invasiveness quality of cancer cells is a principal contributor to these deaths by influencing tumor growth and metastasis. Current methods for evaluating this include seeding cells on bio-gel, capturing images and semi-manually labeling the positions and depths of cells, which are both time-consuming and prone to human error.
This study introduces an automated approach for estimating cell position and depth using Differential Interference Contrast (DIC) and Surface images. Two deep learning models were utilized: Detectron2 for cell detection (bounding box and segmentation) and EfficientNet for depth estimation (per bounding box- cell). Our dataset contained 232 DIC images, which initially included 3,768 labeled cell positions and was expanded to 1,634 images with 26,375 labeled cells through data augmentation techniques. Similarly, the surface dataset increased from 31 labeled cell depths to around 250. The cell detection model was based on Detectron2, evaluated using Intersection over Union (IoU).
The cell depth estimation was based on EfficientNet and evaluated using MAE. Cell detection model achieved high precision and recall with AP50 of 90.8 for bounding box, 88.8 for segmentation, and AR@IoU 0.5:0.95 (large) of 77.6 for both. Cell depth estimation model achieved relatively small MAE- 1.04 𝜇𝑚. The study serves as proof of concept. Since this method reduces manual labor and error, future work may extend it to larger and richer datasets, thus establishing it as invaluable tool for laboratory use.