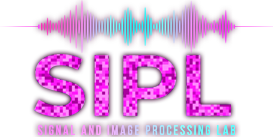
Recent studies have shown that motor learning entails the dynamic reorganization of functional connectivity in the brain’s neural networks.
This work investigates these dynamics by analyzing the layer 2-3 pyramidal neurons of the motor cortex in mice during motor task learning, drawing inspiration from related studies on VTA (ventral-tegmental area) dopaminergic projections and their influence on network
plasticity.
Both prior research and this work employ the diffusion map algorithm with Riemannian distances to effectively reduce the dimensionality of neural activity correlations.
However, this approach often lacks interoperability, leaving the identification of relevant features unresolved. The goal of this work is to propose an analysis pipeline for revealing
the intrinsic sub-networks driving network organization. First, we validate the utility of the diffusion map algorithm in uncovering patterns in neural data by showing that there is a
gradual change of the correlations structure that describes the proficiency level of the mice as described in recent studies and show that the diffusion map algorithm extracts this change in lower dimensions better than linear dimensionality reduction
methods by comparing its ability to predict the proficiency level compared to the other methods. Second, to identify meaningful correlations that contribute to the learning dynamics.
By integrating dimensionality reduction with feature selection techniques, this project seeks to provide insight into the underlying processes of neural network adaptation during learning.