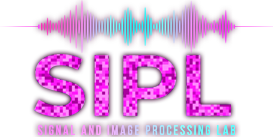
Long-QT Syndrome is an arrhythmia that might cause additional life-threatening arrhythmias. Thus, early detection of the appearance of Long-QT in people exposed to environmental factors, such as drug exposure, is vital.
To avoid unnecessary hospitalization of patients for tracking their heart function, it’s desirable to develop a detection method for finding such cases using mobile ECG devices. These devices usually measure a single lead of the ECG.
In this work, we evaluate several deep-learning-based solutions to detect cases of high-risk Long-QT out of single lead ECG measurements, with emphasis on evaluating the possibility to use mobile ECG devices for such detection. The detection ability was evaluated using Lead-I ECG, which is measured as a voltage difference from both hands.
As part of this work, we implement one solution, named QTNet, and evaluate it on open-access datasets. This solution showed better accuracy in predicting the QT interval and heart rate of a patient out of the single lead ECG in comparison to a classical method algorithm. However, we saw that in order to evaluate the accuracy of detecting high-risk cases of Long-QT, one should work with richer and more balanced datasets, as applying augmentations to the model training set did not improve the accuracy and even harmed it.