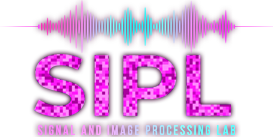
Lexical stress plays a crucial role in distinguishing word meanings and grammatical functions, particularly in minimal pairs (e.g., PREsent vs. presENT). The aim is to propose a classifier for detecting the stressed syllable and understanding the acoustic features underlying the decision. Disyllabic stress minimal word pairs and non-minimal word pairs (e.g., WALlet vs. extEND) were selected, and these words were located in several speech corpora using a forced aligner. A part-of-speech tagging system was used to label each minimal pair’s words as either a noun, which is associated with stress on the first syllable, or a verb, which is associated with stress on the last syllable. In non-minimal pairs, stress placement is unambiguous and consistently follows lexical conventions. A CNN binary classifier was built and trained with focal loss to address class imbalance, achieving a classification accuracy of 92%. Layerwise Relevance Propagation was employed to generate heatmaps on the spectrogram, highlighting the signal regions used in the classifier’s decision-making process. Additionally, acoustic feature, including the fundamental frequency and the first two formants, were extracted to evaluate their impact on the model’s predictions.