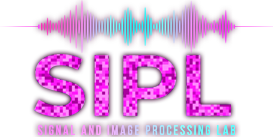
Traffic flow forecasting is a crucial task for urban mobility and intelligent transportation systems. In this work, we explore graph-based deep learning approaches to model traffic dynamics across space and time using real-world datasets from multiple cities. We represent traffic data as weighted directed graphs, where nodes correspond to spatial locations and edges represent road connections, weighted by the average driving speed in different time slots.
We examine different methods, including MagNet Graph Neural Networks (GNNs) and dynamic graph models such as DyRep [2] and Self-Supervised Learning (ST-SSL). Our primary focus is on adapting MagNet to effectively handle weighted spatial-temporal information, evaluating symmetric and non-symmetric adjacency representations. We present our methodology, dataset processing pipeline, and experimental results, demonstrating the potential of graph-based methods for traffic flow forecasting.