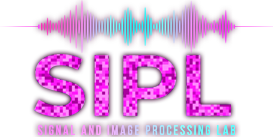
This work focuses on developing a Vision-Language Model (VLM) for identifying the hyoid bone in CBCT images and analysing its position relative to the mandibular plane.
CBCT scans are commonly used in dental clinics but have not been fully utilized for diagnosing Obstructive Sleep Apnea (OSA). The project's goal is to develop an AI-assisted diagnostic tool that provides automatic textual descriptions of the hyoid bone's position, assisting clinicians in early detection.
We adapted the MyVLM (Alaluf et al., 2024) architecture for medical applications, replacing key components with Medical CLIP and LLaVA-Med, which are specifically trained for analysing anatomical structures.
The results demonstrate low accuracy in detecting and describing the hyoid bone. In this article, we explore several strategies for improving the model's performance, including dataset augmentation, prompt engineering, and architectural adjustments tailored to medical imaging. These enhancements aim to increase diagnostic accuracy and support clinical decision-making in the context of CBCT-based analysis.