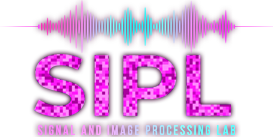
This work investigates the Electrodermal Activity (EDA) signal as measured by the Moodmetric ring, a smart wearable device. The primary goal of the project is to explore the potential of EDA signals for classifying subject mental stress. This is based on the assumption that variations in skin conductance, measured by EDA, reflect changes in an individuals mental and emotional state. We begin with a comprehensive analysis of the physiological basis of EDA, its measurement methods, and various applications. Our methodology includes preprocessing and decomposing the EDA signal into its tonic and phasic components, followed by feature extraction for a binary classification model. This model classifies the EDA samples into two categories: those indicating stress and those signifying a relaxed state. The challenges encountered during the project, such as motion artifacts, EDA signal variability, and individual differences in response to stimuli, are also discussed, alongside the solutions implemented to address these challenges. Finally, we conducted an experiment at the SIPL lab, its samples were analyzed, and the findings demonstrate the efficiency of the Moodmetric ring in stress detection while sampling a motion-less subject. This project contributes to the growing field of wearable technology for health monitoring, offering insights into EDA signal processing and its practical applications for stress management.