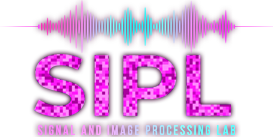
An important task in modern data analysis is to identify a subset of features from a larger pool, which influence the classification result, while keeping a certain error rate under control. This problem appears in many areas of science, genetics, health, decision making, explaining machine learning and many more. For example, finding which genetic mutations are linked to a certain type of cancer, or a doctor wanting to know which of the drugs the patients took really affected the success of the medical treatment.
An algorithm called e-CRT tackles this feature selection problem in a dynamic environment where the sample size is not predetermined. A key advantage of the e-CRT is the ability to work with any machine learning model, where choosing a specific model affects the performance of the e-CRT. In this project, we designed a learning model for this algorithm that aims to improve the performance of e-CRT. This model was built by doing a preliminary examination of the values that we would like to maximize in order to improve the performance of the e-CRT and adding these values to the optimization problem that is focused on during the training. This is how we got a specific learning model that is adapted for integration within the above algorithm and leads to optimal results.