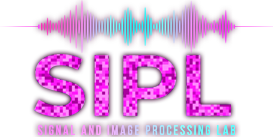
With the expansion of neural network usage, systems based on them have become targets for various manipulation attacks. One of the common types of such attacks is adversarial attacks, which involve adding noise to the incoming signal to the system in order to produce a false outcome (adversary noise addition).
This project focuses on adversarial attacks on speech signals in speech classification systems. As part of the research, neural networks based on the VGG model were trained on two types of speech signal datasets: the first one containing words, and the second containing vowels.
Attacks of varying intensities were applied to the input signals, causing the network to make mistakes. The influence of these attacks on the LPC model of the signals was examined. The research results indicate that the LPC is resilient to these attacks and does not significantly change.