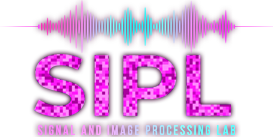
Heart cardiovascular disease is a leading cause of death globally, with over 17 million deaths each year according to the World Health Organization (WHO). Accurate classification of heart sounds is crucial for early detection and effective management of heart conditions. However, this task is challenging due to the complexity of heart sound data, which includes variations caused by low quality recordings and differing physiological conditions. Robust and efficient models are needed for handling such diverse data and improving diagnostic accuracy.
In this work, we propose a machine learning-based solution using Deep Convolutional Networks. The dataset, which includes over 3000 recordings of heart sounds, undergoes comprehensive preprocessing, including segmenting heart sound recordings, applying visual transformations, and augmenting the dataset. Using transfer learning and leveraging advanced image classification networks, we matched the architectures and trained the models to achieve successful classification of heart sounds into two categories: normal and abnormal.
Our experiments yielded high accuracy in heart sound classification. We overcame data limitations, including a relatively small and low-quality dataset, and improved diagnostic potential. Despite these challenges, our approach offers promise for enhancing heart condition diagnoses. This work underscores the potential of deep learning in medical diagnostics, enabling faster and more accurate identification of heart conditions.