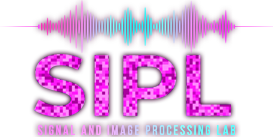
Background noise and reverberation can degrade the quality of speech signals and reduce their intelligibility. Reverberations also reduce the performance of important systems such as hearing aids or voice recognition applications. There are a variety of classic methods for dereverberation of speech signals, but their performance is usually unsatisfactory and not generalizable. In light of this, there has been an increase in recent years in research on dereverberation using modern methods based on deep learning. A common approach to dereverberation using these methods is training a deep neural network to learn the mapping from reverberant speech to clean speech, on the time or frequency domain. As part of this project, we implemented a deep neural network for this purpose, and examined its performance. In addition, we proposed a configuration that uses two microphones, one directional and one omnidirectional, and investigated whether this configuration can be used to improve dereverberation performance.