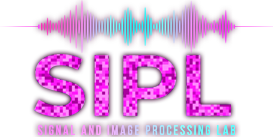
The ECG signal is an important vital signal and plays a critical role in diagnosing heart issues which are lead causes of mortality around the world. The role of ECG analysis in clinical practice is limited by the accuracy of the existing models.
DNNs (Deep Neural Networks) are series of transformations that learn to perform tasks from experience / examples. This approach has achieved great success in many fields in recent years including the medical field, making it a promising approach to replace existing methods of ECG analysis.
In this project we introduce a DNN model which was trained on a dataset of over 1 Million labeled heart beat examples measured by 1 lead and analyzed by cardiologists from different patients and sources. The DNN was trained to detect morphological anomalies in heart beats which then was used in tandem with heart-rate to detect arrhythmias.
The sensitivity and precision in detecting anomalies of beat morphology with our method surpassed the average precision of cardiologists in 4 different classes (PVC PAC BBB N Other) and 5 different heart-rate issues (VT, SVT, Bigeminy, Trigeminy, Normal).
These findings show that Deep Learning can be used to reliably classify morphological anomalies in heart beats for detecting arrhythmias using only a single lead, with performance identical or surpassing cardiologists. This method could be used to reduce the rate of misdiagnosis made by computers and improve the diagnosis done by cardiologists by focusing the cardiologists attention on key areas and prioritizing urgent cases.