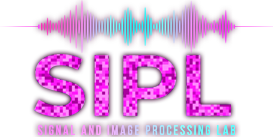
Spinal Surgery is a high-risk procedure with sever potential complications including paralysis and permanent sensory loss. Most of these complications are preventable or can be mitigate using Intra-Operative Neuromonitoring. The field of IONM is rather new, but it is rapidly becoming a standard-of-care in neurosurgery, orthopedics and ENT (ears, nose, throat) procedures. During neuromonitoring of a case, relevant bio-signals are recorded and processed prior to and during the surgery, by which the neurophysiologists can detect pending neurological insults. EEG is one of the most important bio-signals in neuromonitoring, allowing to assess the depth on anesthesia.
In this project, we researched and implemented methods to approximate the depth of anesthesia from raw EEG signals, using machine learning and hand-crafted feature-extraction methods.