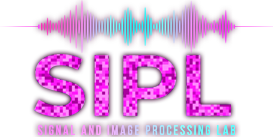
In this work, we dealt with a physiological signal called EDA, the Electrical Dermal Activity of the skin. Under the assumption that changes in an individuals mental and emotional state produce variations in the electrical dermal activity of the skin, we investigated the ability to classify stress based on this signal. During the project, we explored different signal processing and feature extraction techniques. We applied a feature selection algorithm to select the most informative features and to reduce the model's complexity. Based on the selected features, we trained an SVM classifier for the binary classification of stress and relaxation samples. We worked with several datasets on which we tried the processing and classification while trying to reproduce the results of studies from the literature. At our disposal we had an E4 Empatica wristband that measures several physiological signals, among which we worked with the EDA signal. Finally, we planned and carried out an experiment to measure EDA signals using the wristband. In the experiment, participants watched several short videos selected to stimulate stress and relaxation. From the result of the experiment and classification results on the datasets, we conclude that the EDA signal measured by the E4 wristband is noisy but can be used to classify between stress and relaxation.