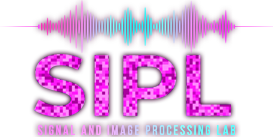
In recent years, the field of artificial intelligence and deep learning is gaining a foothold in almost every field of our lives. In the field of medicine in particular, deep learning has a prominent place in everything related to image processing, information analysis and disease diagnosis. Data acquisition requires skilled and experienced personnel, who are required to perform tasks that are partly Sisyphean and tedious. Also, sometimes it is a matter of working with rare diseases, about which not enough information has been gathered to date. Many medical photographs contain various deformations which make it difficult for the algorithms to achieve optimal detection performance.
Our goal in this project was to improve the diagnosis of pneumonia from chest X-rays with the help of deep learning that is robust to deformations in the images. We examined the use of a new algorithm called Deformable Convolutional Networks which introduced immunity to image deformations in the challenges of classification and segmentation. We modified the algorithm to work on chest X-ray data sets, with the aim of achieving better results, thanks to the robustness to deformations compared to a standard deep learning algorithm. Our results didnt lead to an unequivocal conclusion about the algorithm's robustness to deformations compared to classical networks.