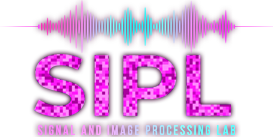
Hypertension is a dangerous Cardiovascular disease that can be measured directly from the blood pressure. Blood pressure changes through the day and night and therefore continuous monitoring of blood pressure can provide more clinical information. However, today's methodologies of blood pressure monitoring are uncomfortable for the user and result in only a single measurement. To address this need, a cuffless, non-invasive, and continuous method was developed in this study using PPG recordings from the PPG-BP database containing 219 patients and 657 PPG recordings. The recordings were pre-processed and underwent a specific screening process to ensure the quality of the signal. Eighty-four features belonging to the PPG waveform and its derivatives and statistical and demographic features were extracted from the remaining recordings. Gaussian process regressor model was built and trained on these features to predict systolic blood pressure and achieved a median absolute error of 6.22 mmHg. The trained model was then applied on a more significant and noisier database with overnight PPG recordings, MESA Sleep, to diagnose hypertension. The MESA recordings underwent the same pre-processing and feature extraction steps as used on PPG-BP. The MESA data was then split into two categories: hypertensive and normotensive patients. However, the results achieved by the trained model in the diagnosis of hypertension were unsatisfactory, likely due to the differences between MESA Sleep and the PPG-BP database.