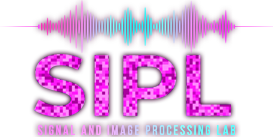
With the large increase in human marine activity, our seas have become populated with boats and ships projecting acoustic emissions of extremely high power that often affect areas of up to 20 square km and more. The underwater radiated noise (URN) level from large ships can exceed 100 PSI and is wideband, such that even at distances of several kilometres from the vessel, the acoustic pressure level is still high with a clear disturbance impact on the hearing and behaviour of marine fauna.
However, while current studies focused on visual observations and signal processing on dolphin whistles for learning about the behaviour of dolphins, a clear statistical proof of such impact of shipping URN on dolphins has yet to be provided.
In this work we developed a methodology to confirm or disprove the theorem that shipping URN impacts dolphins by exploring classifying capabilities of dolphins' whistles in the presence of a vessel. We argue that if such classification succeeds, such an impact exists. We explored the above methodology over a database of tagged acoustic recordings of dolphins whistles and shipping indication taken over a month period in Eilat, Israel.
Through machine-learning classification, we showed that features from dolphin's whistles can be used to predict if a vessel is nearby, thus proving that dolphins are affected by nearby vessels