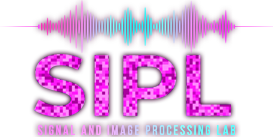
One of the main challenges with diagnosing autism is the lack of a structured method of diagnosis. Today, the way autism is diagnosed is by observing behaviors of the subjects, which develop at a late stage, around the age of 4. This late diagnosis has its toll, mainly a late start of treatment and increased difficulty in integration into general society.
SensPD company, with whom we worked during the project, have a theory that autism can be diagnosed using a cutting-edge method. They state that using auto-acoustic emissions (OAE), a distinction can be made between subjects on the autistic spectrum (ASD, autistic syndrome disorder) and subjects not on the spectrum (TPD, typically developing).
This project is made up of two parts. Firstly, we processed the data using Fourier analysis tools to verify the results achieved by SensPD. This work included pre-processing of the signals, creating spectrograms and analyzing them, and raising conclusions regarding the features of the data at different frequency ranges.
In the second part of the project, we utilized unsupervised learning tools to analyze the signals. We used a tool called Diffusion Maps. As a part of this analysis, the signals were pre-processed, and developed using the diffusion maps algorithm in order to create groupings of points in the 2-D plane.