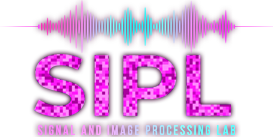
Maintaining the security of maritime space is an important security interest of every country. In this project, we propose a machine-learning and deep-learning-based method for the classification and identification of ships based on signals transmitted by their radars. We represent the signals with a unique representation in the time-frequency domain and use it as an image. Neural networks have shown themselves to be very efficient in image classification, thus, the idea of using images stands to reason, and we indeed achieved good results in the classification stage.
Another challenge we addressed in our work was Open World Recognition, which is the problem of identifying and classifying new ships, i.e., ships from classes that did not appear in the training set. We achieved significant improvement over previous work on the mission of recognizing unknown ships (Open Set Recognition), with a focus on reducing false alarm rate, using anomaly-detection-based algorithms. We also developed an unsupervised learning-based algorithm that provides a good solution to the problem of learning new unknown ships on inference time without re-training. We provide a complete solution to the problem that can form a good basis and infrastructure for further future work.