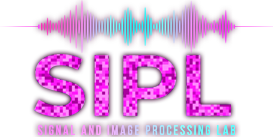
The goal of this work is to design a method for performing voice conversion between two speakers. The method employs deep learning techniques, particularly autoencoder architecture, to convert the source speakers voice into the target speakers voice while preserving the source speakers linguistic content. The baseline model architecture is VC-AGAIN. This model uses a one-shot approach. In this approach, it is sufficient to receive in the inference stage a single speech signal from the source and target speakers, on whom the system has not been trained, in order to perform voice conversion. We proposed several changes to the baseline model, including adding the pitch frequency as a model input, which enriches the learning phase and improves feature extraction performance; and replacing the vocoder with HiFi-GAN, a new vocoder that may outperform the baseline vocoder. By conducting subjective tests, we were able to evaluate the performance of our proposed models. We conclude that the model with the pitch frequency added outperforms the baseline model in both the naturalness and similarity test. Moreover, switching to the HiFi-GAN vocoder has degraded performance.