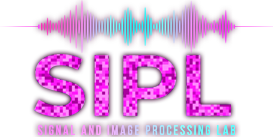
The goal of Brain-Computer Interface (BCI) systems is to enable paralyzed individuals independent control of external devices using the operators brains activity. As many BCI systems are based on electroencephalographic (EEG) signals to avoid invasive procedures, a consistent challenge is to design more robust and reliable classifiers for these signals. Although BCIs are intended for individuals who cannot move, oftentimes classifiers are calibrated on signals from healthy subjects executing movements. In this project, we simulate the real-world scenario by testing on signals from a new subject (unseen in training) imagining movements. We show training on actual, imagined, or both signals, leads to erratic classification results. We propose this is because the signals lay in different domains, and apply a domain adaptation technique, Frustratingly Easy Domain Adaptation (FEDA), to adapt a classifier to the clinically-relevant imagined domain. Using FEDA, we significantly enhance classifier performance by 8% (from 25% to 33%) on a difficult four-class discrimination task.